Harnessing Unstructured Data to Gain Insights
- March 23, 2023
- Posted by: Aanchal Iyer
- Category: Data Science
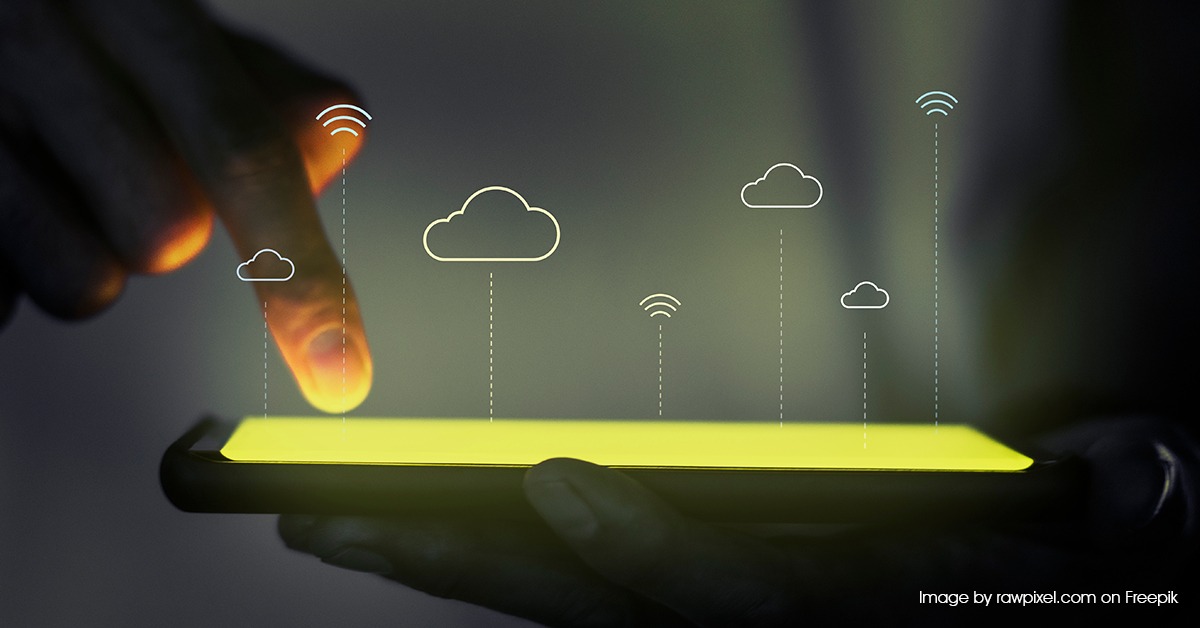
Introduction
Organizations use data to gain insights which is a well-known tactic within the corporate world. While this idea is quite easy to understand, executing it may prove to be a bit of a challenge. There are various reasons for this, including the lack of qualified people to perform data analytics, scarce toolsets as well as incorrect assumptions.
However, the biggest obstacle for success comprises not having a complete view of the entire data set. It is quite tempting to create data warehouses from prevailing databases and use the data results for analytics. However, the problem with this approach is that it depends too much on structured data. Unstructured data, for example, the one that is found in documents and e-mails is not often taken into consideration. This impacts the accuracy of the data analytics process.
What is Unstructured Data
Structured data includes text or numbers that can fit into the fields of relational database management system (RDBMS) such as Microsoft SQL Server or Oracle. It takes the form of a database’s columns and rows. Unstructured data comprises information that does not exactly fit into an RDBMS. It lacks uniformity. For example, a customer database comprises a structure including the first name, last name, phone number and so on.
It can be found in a PDF document, social media post, or an e-mail thread. It is numbers and text and perhaps sounds, images, and video.
Why Analyze Unstructured Data?
Unstructured data is more difficult to collate, process, search and analyze than its structured counterpart. Yet, 80% of data is unstructured, and contains a lot of hidden value. For example, much of what advertisers term as “brand sentiment” is available in unstructured data.
You may be able to identify a problem in customer loyalty using structured data sets, such as sales ledgers. If customers make fewer repeat orders, then that indicates a brand sentiment problem. One can identify negative brand sentiment if social media posts reveal the actual comments on the product. To view those comments, you have to analyze the unstructured data.
Another gripping reason to analyze unstructured data is for data classification. Data classification is the process of detecting and then labeling data according to classifications, such as “confidential,” “intellectual property” or “IP,” “personally identifiable information” “PII” and so on. Data classification is foundational for compliance and data security. It is nearly impossible, after all, to be able to protect data if one does not know what it means.
More serious data security programs prioritize defending an organization’s most valuable and delicate information. To be able to do this, it is essential to view all possible data sets. Doing this means analyzing unstructured data. For example, an organization may apply a premium charge to protect its patents. However, there is a chance that the information supporting your patent applications is spread out across your enterprise. Documents in file drives and cloud volumes could comprise rich intellectual property, such as research reports and engineering drawings that competitors could steal.
Conclusion
Unstructured data is a major part of an organization’s data analytics strategy. It needs to be built into data security and compliance efforts. The consequences of ignoring are potentially quite severe. A modern enterprise search solution is a key element in any project that sets out to discover, classify and analyze unstructured data.