Generative AI in Healthcare: From Diagnosis to Personalized Treatments
- January 16, 2025
- Posted by: Aanchal Iyer
- Category: Artificial Intelligence
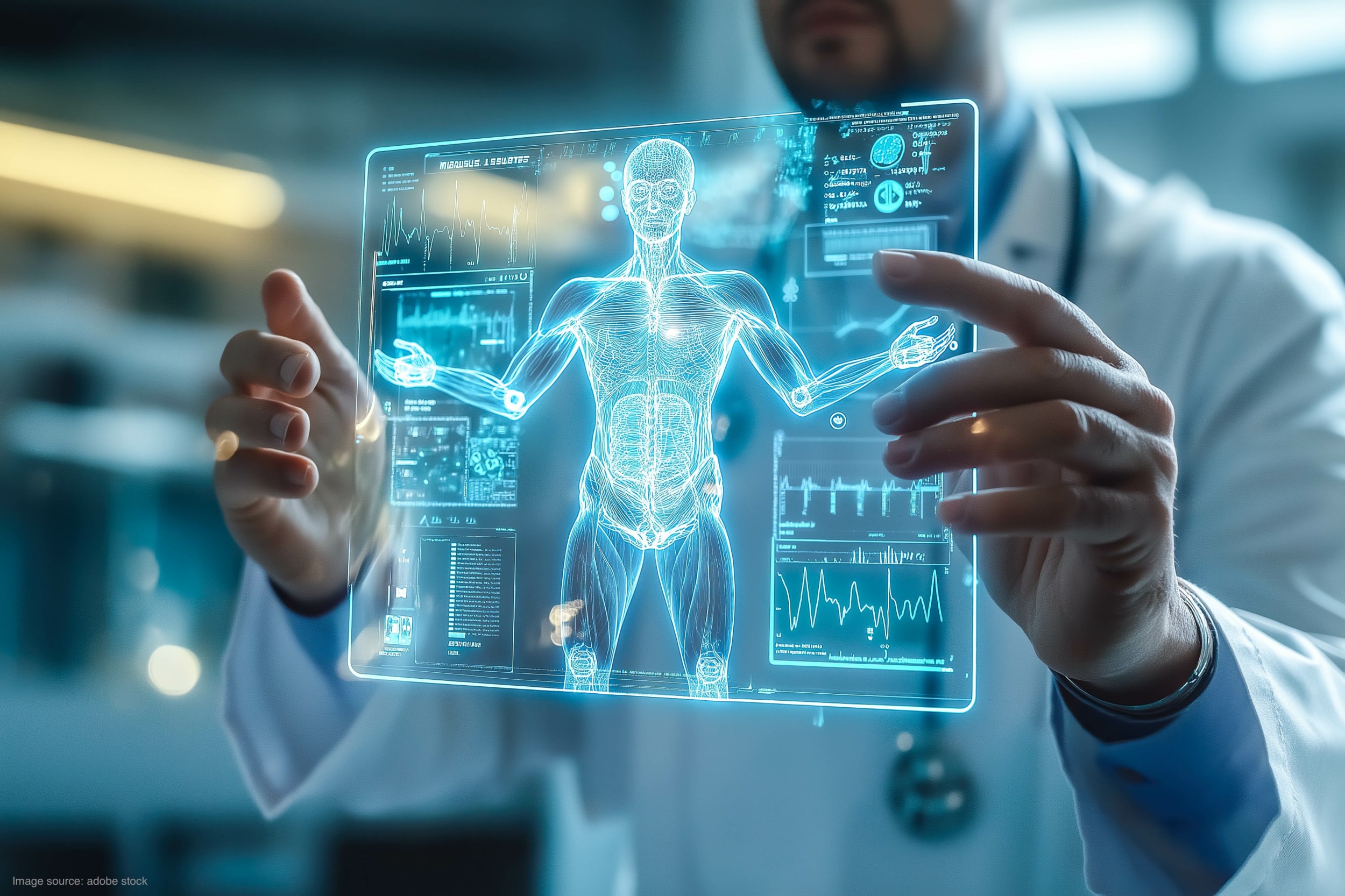
Generative AI has brought a new era of possibilities in various verticals across industries. This revolutionizing technology can transform how we look at and try to resolve complex problems by providing path-breaking solutions and innovative outcomes that were once unimaginable.
Like most industries, the healthcare landscape is at the point of transformative progress backed by Generative AI. Machine Learning (ML) algorithms are continuously evolving and reshaping the different aspects of the healthcare industry, surpassing the boundaries of traditional approaches.
From diagnosis and treatment to discovering drugs and custom-made medicine, Generative AI is all set to transform how healthcare professionals view complex medical challenges.
Applications of Generative AI in Healthcare
It has powerful tools for analyzing medical data, helping them perform accurate diagnoses, and creating personalized treatment plans. Some of the critical impact areas are :
Drug Discovery and Development
Generative AI can forecast the properties of potential drug candidates, create new molecular structures, and leverage existing molecules to enhance their safety and efficacy. This significantly minimizes the time and cost of drug development.
Medical Imaging
Generative AI for healthcare has the power to boost medical imaging techniques by creating high-quality images, regenerating missing or corrupted data, and helping with image analysis and segmentation. This helps clinicians make accurate diagnoses and treatment decisions. Once applied to CT images, it can minimize the amount of radiation required – a huge benefit to patients. Generative AI also creates 3-D holographic images from CT and MR scans, which significantly helps surgeons during complex procedures.
Mental Health Assessment and Intervention
AI models, such as ChatGPT, can help design chatbots and virtual assistants for mental health support, triage, and therapy. These tools help bridge the gap in mental health care by providing scalable and accessible solutions.
Personalized Medicine
The technology can analyze large-scale patient data to identify relationships and patterns that can guide customized treatment plans, while considering genetic predisposition, lifestyle, and environmental factors.
Digital Avatars
Generative AI can design realistic virtual patient populations, that can help test and enhance medical interventions, perform clinical trials, and train healthcare professionals. This is considered as the final frontier of Generative AI in patient care.
Integrating AI with Healthcare Data
A huge benefit of Generative AI is that it can process tonnes of data and find humanly impossible solutions. Integration between healthcare data and Generative AI models is key to better patient care, rearrangement of workflows, and identifying valuable insights from complex datasets.
Electronic Health Records (EHRs)
These are digital records that contain comprehensive patient information, including medical histories, lab results, and treatment plans.
AI algorithms analyze EHRs to find patterns and trends (including text and image data) that may not be clear to human doctors right away. This analysis can help predict potential health risks and suggest personalized treatment options.
Data Analytics and Patterns
AI can process large volumes of healthcare data to detect patterns and make predictions. Advanced analytics techniques can detect correlations between different health factors, which results in improved diagnostics and treatment strategies. Machine learning algorithms can also analyze diverse datasets, including:
- Patient demographics
- Genetic information
- Lab results
- Medical histories
- Imaging studies
By examining these datasets, AI systems can identify subtle patterns that can indicate early signs of diseases or forecast patient outcomes. This capability helps healthcare providers deply proactive intervention measures as well as customized treatment plans.
Ethics, Privacy, and Regulation
There are significant concerns around fairness, data protection, and governance with regards to Gen AI. Addressing these issues is crucial to ensure responsible implementation and maintain public trust in AI-powered medical technologies.
Addressing Bias and Equity
AI systems can elevate existing biases within healthcare data and practices. To prevent this, developers need to leverage diverse training datasets. Regular audits of AI outputs can also help identify and correct biases.
Moreover, if we need to develop AI ethically, then we need multidisciplinary teams that include clinicians, ethicists, and patient advocates. This ensures that AI tools take into consideration different requirements and perspectives.
Also, ensure equal access to AI. Healthcare must should implement AI solutions that serve diverse patient populations, not just those with the most resources.
Patient Privacy and Data Security
Another grave area of concern is patient privacy. Strict data anonymization and encryption protocols should be enforced. AI systems often work on large datasets for training and operation. Healthcare organizations should deploy robust cybersecurity measures to mitigate data breaches.
Informed consent processes need updating to account for AI use. Patients need to understand how their data may be leveraged in AI systems and that they can opt out.
Regulatory Considerations
Globally, governments are looking at ways to best regulate AI. The FDA is developing frameworks to manage and evaluate AI-based medical devices. This includes guidelines for continuous monitoring and updating of AI algorithms. However, clear guidelines are needed to determine responsibility when there are medical errors due to AI. Moreover, international cooperation is crucial to create harmonized AI regulations. This ensures consistent standards and drives global development and deployment of healthcare AI.
Challenges and Limitations
Generative AI in healthcare has significant hurdles in technical capabilities and market acceptance. These challenges have an effect on both the technology’s efficiency and its adoption in medical settings.
Moreover, most organizations hesitate to deploy Generative AI models such as GPT-4 and others as they do not want to deal with AI hallucinations – when models create wrong data, which can pose a serious risk in medical contexts. However, various measures can be deployed to prevent AI hallucinations.
Also, high implementation costs and the requirement for specialized infrastructure discourage many organizations from adopting Gen AI.
Final Thoughts
As Generative AI advances, we can expect to see even greater integration of AI technologies into healthcare systems worldwide. This includes advancements in medical image analysis, virtual assistants, and personalized medicine, among other areas.
Effective integration of Generative AI into healthcare relies on carefully balancing its potential benefits with inherent risks. Leaders must thoroughly assess each potential application, comparing its advantages against potential hazards.
Aretove’s expertise in Generative AI can significantly transform the healthcare landscape by enhancing diagnostics, streamlining clinical workflows, and enabling personalized treatment. Their ability to harness advanced AI models can lead to more accurate medical image analysis, improved patient data handling, and better predictive capabilities, ultimately contributing to better patient outcomes and a more efficient healthcare system. As the demand for AI-driven innovation grows, Aretove is well-positioned to support healthcare providers in leveraging Generative AI for a smarter, more responsive, and patient-centric future.