Combating Shadow Data
- November 21, 2022
- Posted by: Aanchal Iyer
- Category: Big Data Analytics
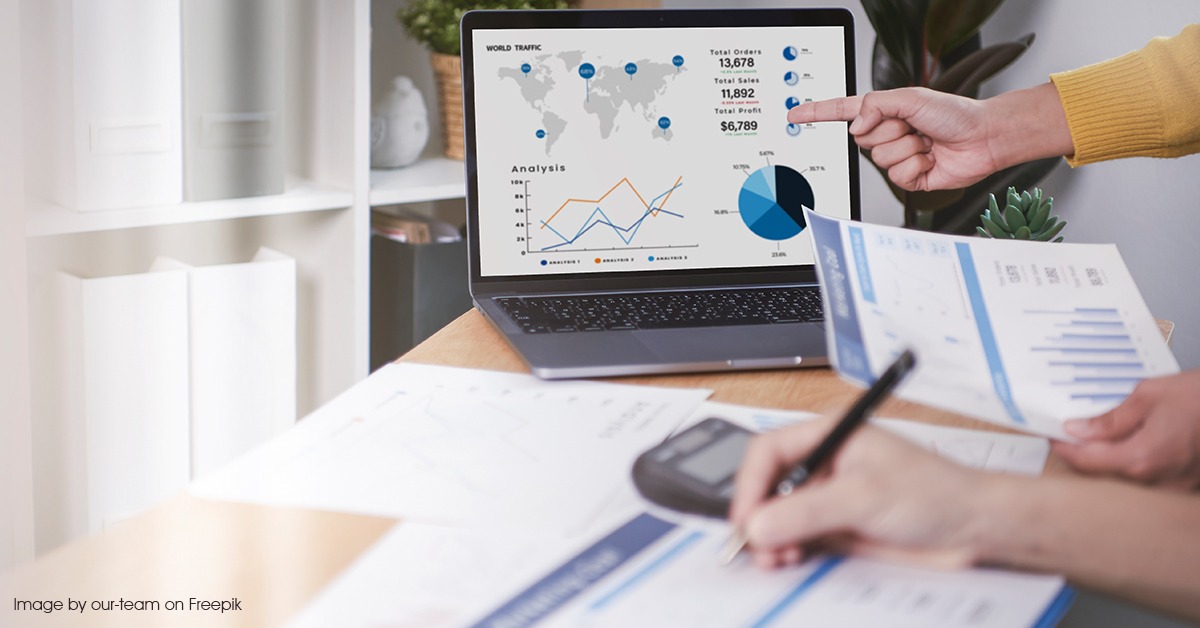
Introduction
Shadow data is any confidential or sensitive data that escapes from systems/devices or cloud, inadvertently or intentionally. Examples of shadow data are:
- Employees share confidential information by mistake, assuming that the data isn’t sensitive.
- Data that has been unknowingly abandoned or duplicated.
- Data that lies somewhere in an enterprise, but is not a part of the enterprise-wide data management platform.
Why is Shadow Data a Significant Problem in the Cloud?
With most organizations moving to the cloud, the concerns regarding shadow data are only increasing. Team-sharing and cloud-based collaboration apps, such as Google Drive, Office365, and Dropbox are all susceptible to shadow data threats. Other than these file-sharing apps, users can store sensitive data in CRM apps, video-sharing devices, and online tools. It is also very easy for data and R&D teams to develop and move data in the cloud while building new applications or models. All these are issues concerning shadow data.
Shadow Data can Put Organizations at Risk
As shadow data is the data that the organization’s administrators are oblivious to, the threat to the business rests on the data sensitivity. Employee and customer data that is not secured properly can result in compliance violations, especially when health or financial data is at risk. And then, there is also the threat that confidential data can escape.
How are Dark Data and Shadow Data Different from Each Other?
Dark data are information assets that businesses collate, process, and then store during regular business processes but generally fail to use this data for other purposes. Organizations often start keeping dark data as a part of compliance requirements. It can comprise past financial or employee information, confidential intelligence data, transaction logs, internal presentations, emails, download attachments, and also surveillance video footage. Dark data is data created by a user’s daily digital interactions within the course of general business processes. In contrast, shadow data is either purposely created outside an organization’s IT infrastructure to be able to leverage cloud and SaaS applications or unintentionally by organizational over-sharing. Either way, shadow data always presents security risks.
Three Keys to Securing Shadow Data
- Visibility is Key. The goal for the security teams needs to be to identify each cloud-managed environment and SaaS application in which the organization may contain sensitive data. One cannot apply security controls to data within repositories that is not visible.
- Data Classification and Discovery. One must be able to identify all data available in the repositories and categorize sensitive data to apply security controls to it. The best way to do this is to roll the data repositories into an individual source and receive dashboard access to see what is going on across all the data sources to identify anomalous behavior rapidly.
- Control Data Access Privileges. An effective analysis of irregular behavior is very effective at identifying malicious user activities. Machine Learning (ML) algorithms can regulate access for privileged users and send regular alerts if preventive measures are not followed. ML analytics can also identify the data that is business-critical and check if a privileged user can access that data.
Conclusion
Digital transformation and the drive to leverage cloud has resulted in huge volumes of shadow data. An average organization cannot manage such a huge volume of shadow data effectively and keep it secure. Having full data observability enables one to understand where your shadow data is being stored. Doing so results in a secure environment along with a smarter and faster decision-making process across the organization.