How Predictive Modelling Helps In The COVID-19 Epidemic?
- September 13, 2020
- Posted by: admin
- Category: Predictive Analytics
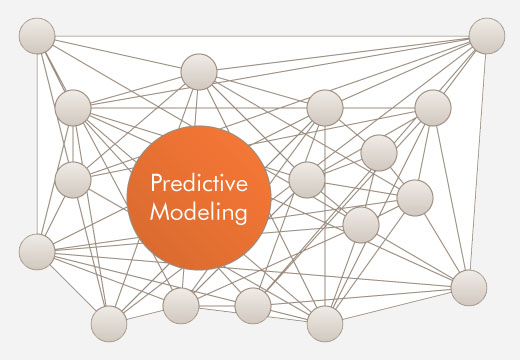
Introduction to Predictive Modelling
WHO announced a Public Health Emergency of International Concern on Jan 30, 2020, which was almost a month after COVID-19 was acknowledged in Wuhan, China. By then a number of computational and mathematical models had already announced about the possibility of the Severe Acute Respiratory Syndrome Coronavirus 2 (SARS-CoV-2), which could lead to a pandemic situation with dreadful consequences globally. When a novel pandemic of such magnitude surfaces, predictive modelling is essential to prepare and respond with measures for the public health. Models are associated to the data available and this brings about insights into concealed variables, such as the occurrence of infection and the amount of cryptic transmission. These models enable the examination of hypothetical interventions and counterfactuals. There have been incredible developments in mathematical epidemiology, however predictions about epidemic outcomes are never accurate.
Predictive modelling is highly valued where assumptions are concerned. The variables to be assessed are defined clearly and policy makers or researchers using the model outputs have a clear understanding of the limitations of this method. In fact, there has been a demand for national disease-forecasting centers with the requirement of educating policy makers at all levels on how to integrate predictive modelling into the decision-making procedures.
Diverse data sets are mandatory to get insights with predictive modelling, and these data sets are often flawed, specifically during the critical period when the epidemic starts. This initial period is when, not much is known about the epidemiology or the medical features of the disease. For example, widespread genomic data and clinical case counts were combined with extensive records of human mobility and behavior. These were utilized using predictive modelling, where digital information sources were widely deployed.
Predictive Modelling in the COVID-19 Epidemic
After the first reported case of COVID-19, predictive models not only expected the pattern of international spread, but also measured the extent of the epidemic in China. The predictive models used in Wuhan also indicated that there were more cases of the virus present than reported.
Detecting the potential inconsistency between reported cases and the actual disease burden provided early warnings to the international community. Next, data-driven computer simulations and statistical modelling gave exact estimates of the global epidemic dispersion. This, in turn quantified the role of social distancing in China and decrease in international travel with regards to the spread of COVID-19. Also, the drastic social measures enforced in China, and later on in many countries, resulted in a strong variation in human behavior.
Estimates on the infection fatality rates generated by early studies of travelers led to the later efforts to characterize the unidentified epidemic burden. This was achieved by utilizing different modelling approaches that associate mortality to an unknown epidemic occurrence. As the pandemic advanced analyses based on mobile phone records offered vital support to public health valuations across the various stages of lockdowns deployed across the world.
Google Scholar states that predictive modelling is not being used as much as it should have. However, nearly every hospital, business, state, and national government has been warned with COVID-19 forecasts. This disconnect between the small but fast-growing science around outbreak forecasting and its now widespread application creates a difficult situation for clinicians, researchers, and policy makers.
Conclusion
Predictive modelling has proved its value as it did predict that a global pandemic was most likely to occur and this would have severe consequences on the human health if strong public health measures are not available to limit human contact. As a society, we must leverage the role of digital health to fight against current and future pandemics.