How Valuable is Data Observability
- February 23, 2023
- Posted by: Aanchal Iyer
- Category: Data Science
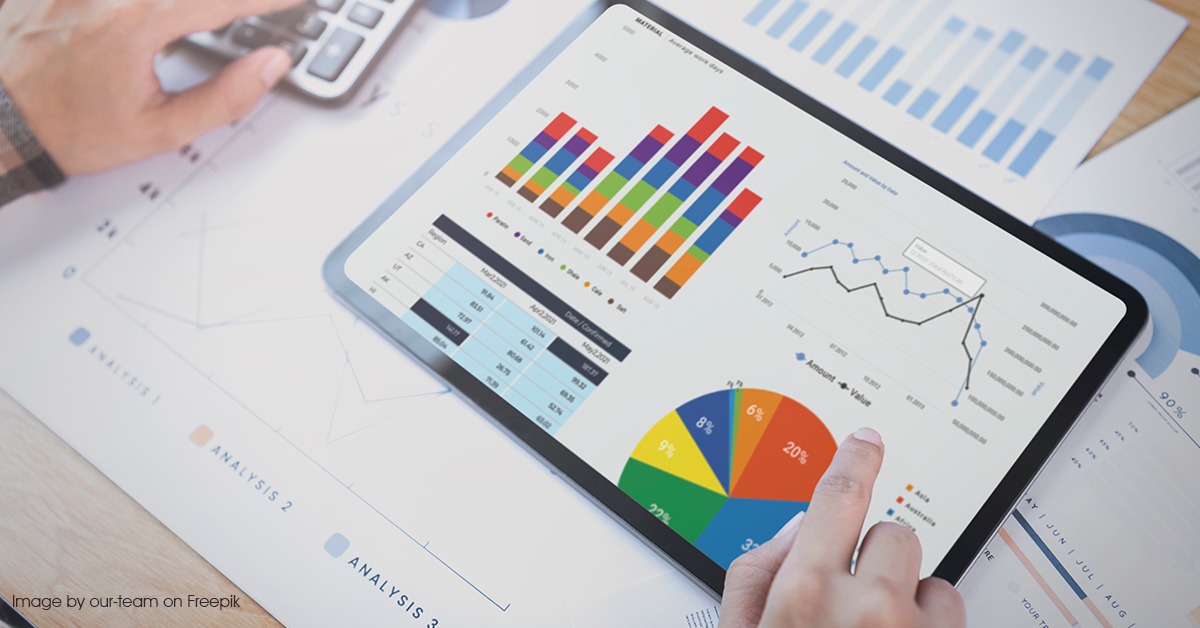
How Valuable is Data Observability?
Introduction
Data is extremely vital, it is in fact the new fuel. All industries currently depend on data to be able function. It is very important that the data being used is clean, precise and error-free. This is exactly where the concept of data observability comes into play. Data Observability is the process of diagnosing the health of the entire data value chain. It is an umbrella term that comprises workflows and technologies that enable one to identify, debug, and fix data issues. Data Observability detects data inaccuracies and helps one determine the main cause of the problems. It also suggests proactive measures to make systems reliable and efficient.
Why Data Observability is Important
The following are the reasons why data observability is important:
Data Observability makes data trustworthy:
Although machine learning algorithms and data insights are very useful, erroneous data can have significant repercussions. Data Observability enhances the confidence in enterprises when making data-driven choices.
Data Observability helps timely delivery of quality data for business workloads
Every organization has to ensure that data is easily accessible and in the proper format. Data scientists, data engineers, and data analysts depend data to carry out their business operations. Data observability maintains the quality, consistency and dependability of data. It offers companies a 360-degree perspective of their data ecosystem. This in turn enables the companies to identify and correct issues that may lead to a breakdown in the data pipeline.
Data observability helps identify and resolve data issues
Data observability identifies the circumstances which met have a severe impact on an organization. This can provide context and relevant information for root cause analysis.
How is Data Observability Different From Data Monitoring?
Data monitoring analyses data in the pipeline with the aim of detecting poor quality data and taking steps to correct it. Data monitoring tools look for data that is inaccurate, incomplete, or does not adhere to defined standards.
On the other hand, data observability does more than basic data monitoring. It examines not just the data quality but also its schema and lineage to offer insight into why it is as it is. It adds the question “why?” to the questions bought up in data monitoring. In fact, data observability can allow for more efficient and accurate data quality management. While data monitoring looks for pre-defined issues, data observability helps identify new and evolving issues.
Pillars of Data Observability
There are 5 key pillars of Data Observability that depict the health of data. They are as follows:
Freshness
Freshness revolves around if the data is up-to-date, which means whether the data includes all the changes without any gaps in time. If the data is not fresh, then this can break data pipelines resulting in various gaps and errors in data.
Distribution
Distribution concentrates on understanding the field-level health of data, that is if the data is within an accepted range. It checks for any gaps within the expected data value and actual data value.
Volume
Volume refers to the amount of data in database. This indicates whether the amount of data being fed meets the expected thresholds. It helps in giving insights into the health of your data sources.
Schema
Schema refers to the data structure supported by the database management system. The schema of the tables in a database should always be in accordance to the way the DBMS complies to.
Lineage
Data lineage identifies the upstream sources and downstream consumers that are affected when the pipeline breaks.
Conclusion
Data Observability is essential for an organization to become data-driven. With the advancements in Data Observability, data governance strategies and data quality frameworks are now much more actionable.