Industrial Data Ops Ecosystem- Key Principles
- March 30, 2022
- Posted by: Aanchal Iyer
- Category: Data Science
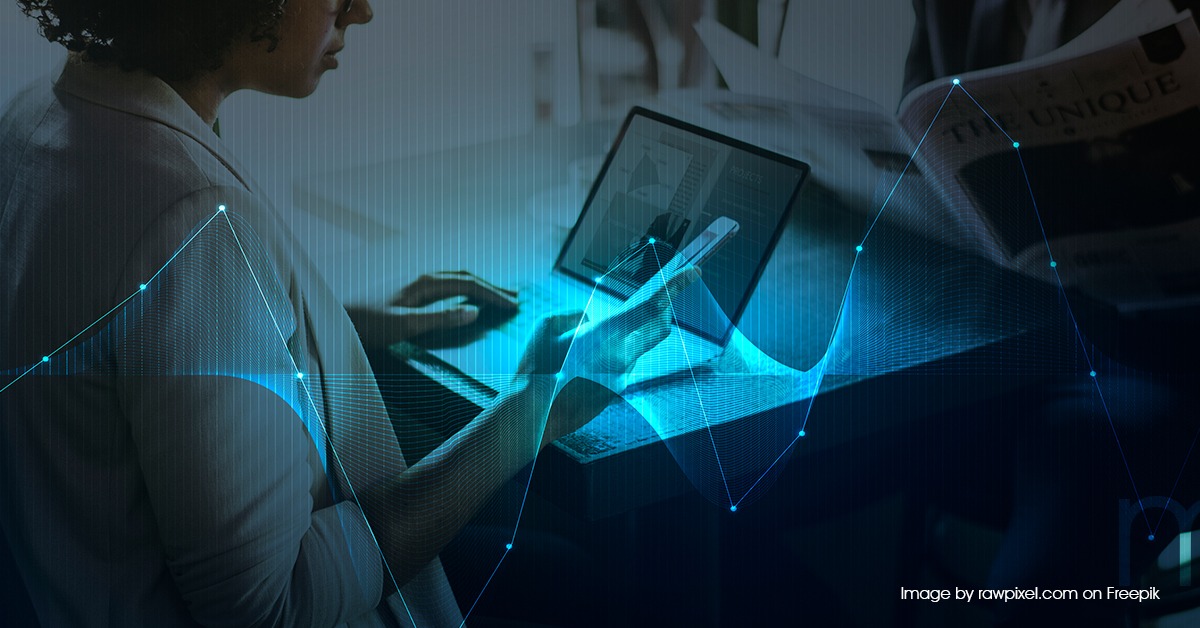
Key Principles of an Industrial Data Ops Ecosystem
Introduction
Industrial Data Ops is turning into a driving force in industrial transformations, helping with the acceleration of digital maturity, enabling data teams to offer more digital products, and realizing more operational value at scale.
There is a consistent pattern of key principles of an Industrial Data Ops ecosystem that is in contrast with the traditional “single platform,” “single vendor” approaches supported by vendors, such as Teradata, Palantir, Oracle, IBM, Oracle, and others. An open approach is difficult, but also more effective in the long term. It represents a winning strategy for CDOs, CIOs, and CEOs who believe in increasing the reuse of quality data in an enterprise. This Data Ops platform also avoids the simplified trap of writing a massive check to a single vendor.
Principles of the Data Ops Ecosystem
There are specific principles of an Industrial Data Ops ecosystem in a large enterprise. A modern Data Ops ecosystem/infrastructure should be:
-
Open
: The best way to describe the Data Ops ecosystem is to talk about what it is not. The main characteristic of a modern Data Ops ecosystem is that it is not a solitary proprietary software artifact or small group of artifacts from a single vendor.
-
Take advantage of best-of-breed tools
: Closely related to having an open ecosystem is adopting best-of-breed Data Ops tools and technologies. This means that each key component of the system is built for a specific purpose, offering a function that is the best available at a practical cost.
-
Highly automated
: The scope and scale of data in an enterprise have exceeded the capability of human effort to organize and move data. Automating the Data Ops architecture and infrastructure and using the principles of highly engineered systems is critical to keep up with the pace of change in enterprise data.
-
Use Table(s) In/Table(s) Out protocols
: The next logical questions to ask if you adopt the best of breed tools are, “How will these various tools/systems communicate? And what is the protocol?”. Table(s) In/Table(s) Out is the key method that should be adopted while integrating these tools and software artifacts. Move or share the tables using the various methods described under Data Services
-
Track data lineage
: As data flows through next-generation data, it is important to properly manage this lineage metadata to safeguard reproducible data production for Machine Learning (ML) and analytics. Having more lineage/provenance for data enables reproducibility that is critical in data science practices or teams.
-
Feature deterministic, probabilistic, and humanistic data integration
: When bringing data together from dissimilar silos, it is appealing to depend on traditional deterministic approaches to engineer the arrangement of data with ETL and rules.
-
Combine both federated and aggregated methods of access and storage
: A healthy next-generation industrial Data Ops ecosystem embraces data that is both federated and aggregated. Over the past 40-plus years, the industry shuffled between aggregated or federated approaches to integrating data.
-
Process data in both streaming and batch modes
: The success of Kafka and similar design patterns has proved that a healthy next-generation data ecosystem comprises the ability to simultaneously process data from source to consumption in both streaming and batch modes.
Conclusion
Industrial Data Ops is a necessary and crucial tool to make digital transformation possible in asset-intensive industries. It demonstrates how to link data from the OT and IT sources to the consumer. Appropriate tips that empower organizations of all sizes to utilize industrial Data Ops are also provided. It is a significant driver of change that will take the industry forward.